I. Session Summary
The conversation with Matt Botvinick revolved around the history and pivotal moments in AI over the last 20 years. It covered the research and innovation at DeepMind, including the development of large datasets, the emergence of deep learning, and the contributions of key researchers. We also discussed the current capabilities and limitations of large language models (LLMs), how the AI landscape is shaping, and implications for founders.
Matt Botvinick’s Background
- Matthew Botvinick is Director of Neuroscience Research at DeepMind where his work straddles the boundaries between cognitive psychology, computational and experimental neuroscience, and artificial intelligence.
- Dr. Botvinick is an Honorary Professor at the Gatsby Computational Neuroscience Unit at University College London. He completed his undergraduate studies at Stanford University in 1989 and medical studies at Cornell University in 1994, before completing a PhD in psychology and cognitive neuroscience at Carnegie Mellon University in 2001.
- He served as Assistant Professor of Psychiatry and Psychology at the University of Pennsylvania until 2007 and Professor of Psychology and Neuroscience at Princeton University until joining DeepMind in 2016.
Matt on Chat GPT, OpenAI, and the race to AGI
“It wasn't like OpenAI had some sort of special wizardry that was hard to replicate. They were just working on a recipe that the competitors hadn't organized themselves to apply it. It was clear when the competitors decided to focus on it, they caught up and overtook them - as Gemini (Google) and Claude (Anthropic) now outperforms GPT4 (OpenAI).”
History of AI and the key breakthrough moments
Deep learning has been around since the 1980s, starting out as part of cognitive science and neuroscience. Both Matt and Denis (founder of DeepMind) did their PhD in Neuroscience and Computational Neuroscience - which is where they started focusing on deep learning.
In the 1990s and early 2000s, deep learning went out of fashion and was considered a dead end by many researchers in the machine learning community. Then there were a few key moments that revived excitement:
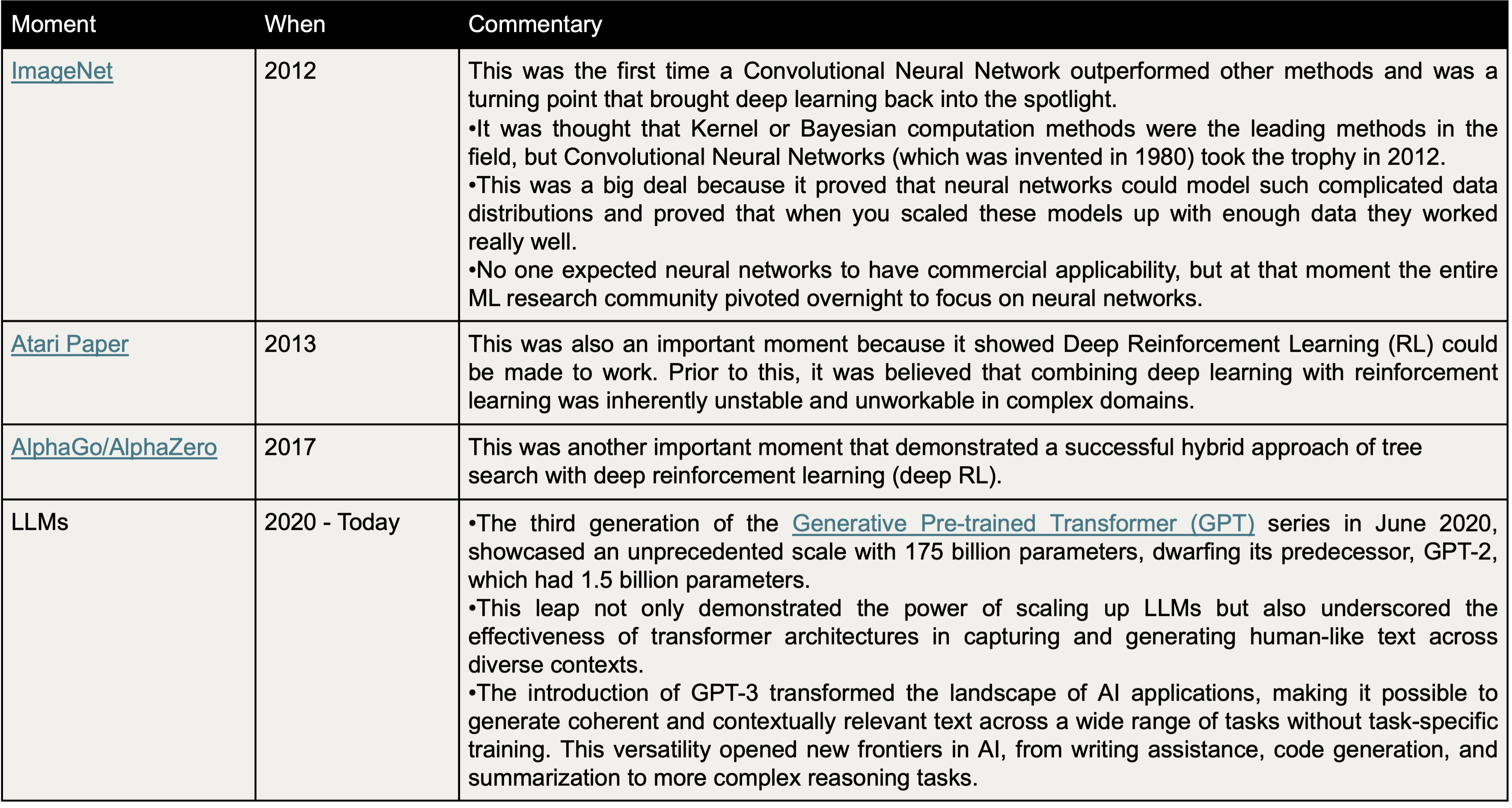
Google’s Blindspots: Technical beliefs and culture
Google’s Technical Blindspot
- Matt was part of DeepMind’s central research planning committee and closely followed the launch of GPT 2 and GPT 3. Prior to GPT 3, large language models (LLMs) were not taken that seriously because DeepMind’s history was deeply rooted in solving AGI via deep reinforcement learning (DRL)
- DRL focuses on training agents to achieve specific goals within defined environments, optimizing actions based on a given reward structure in a trial-and-error manner. This fundamentalist approach to solving intelligence by understanding foundational learning processes was believed by Demis Hassabis (DeepMind CEO) to be how human intelligence works which is why DeepMind stubbornly fixated on it so much.
- Matt points out that it was believed that training with human reinforcement learning was “cheating” because training on human data was not solving intelligence - it was "copying intelligence".
- On the other hand, OpenAI’s mental schema for deep learning was supervised learning. Supervised learning focuses on using large labeled data sets to predict outcomes (i.e., learning from provided input and output data vs. experience).
- LLMs use this approach. With vast amounts of text data, LLMs learn to predict the next word in a sentence, thereby gaining understanding and generating human-like language across a broad range of topics and tasks, aspiring towards a general comprehension and generation capability that isn't tied to specific goals and tasks. This difference in philosophy meant Google initially was caught by surprise with the release of ChatGPT
Origin of Google’s Technical Blindspot
- During our discussion, Matt Botvinik and Barry McCardel (co-founder and CEO) discuss the irony of how Google was not the first to capitalize on the rise of LLMs despite having published the Transformer paper (“Attention is all You Need”). They go on to argue that humans are not born into a world with pure RL-like data, since most concepts and language are taught to us early on - akin to “supervised learning”.
- This goes against what Demis seemed to believe was the right approach to solving intelligence. On top of this, Matt points out you are constantly challenged in Machine Learning research to remain focused and not chase the shiny new approach. Both of these combined to create DeepMind’s blindspot.
Matt and Barry’s (CEO at Hex) conversation on the origin of Google’s Blindspot
Barry
“I am very confused as to where Google’s blind spot came from? It makes sense to me that in something like a game of Starcraft with a well defined set of rules and a game engine re-enforcing these rules, that a system trained purely on self-play with RL would work and that supervised training seemed like “cheating”. However, with Language, it's hard for me to imagine that you could train a system with “self generated speech”
Matt
“Ironically, there were a number of projects at DeepMind aimed at learning language from scratch - i.e: multi language RL systems that would let agents figure out how to create language from scratch but as far as I am aware of, none ever worked.
It would be interesting to apply “psycholinguistic comparisons” to the language created by these agents to see if they came up with a language that followed Chomsky’s grammar rules or something, etc. but it was clear that these agents wouldn’t re-invent a language like English or Chinese via this approach.
The basic and fundamental point here is that when we come into the world as babies we’re not exposed to pure RL-like data. There is content that we are supposed to imitate and learn (similar to supervised learning) so I feel like this was a miss at Google heavily influenced by Demis’s fundamentalist views towards intelligence which were very influential early on.
I think in every field, but in machine learning, in my experience, more than anything else that I've ever encountered, like you are constantly challenged not to go chasing shiny objects, right? Like, every week. There's some sort of optimization you have to do there, which is like knowing when thresholds have been passed. I don't know how you get that tight. But I will say, I was pretty frustrated for about a year at DeepMind because I did feel like people were just kind of stubbornly refusing to see that the paradigm was shifting.”
- However - the success of LLMs (specifically GPT3) has shown that the "universe of language" and the "universe of human concepts" may be smaller than previously thought given that with large enough models trained on large enough data sets, you can build systems that seem to be intelligent.
Matt on the rise of LLMs
“The universe of language is smaller than I thought and the universe of human concepts is smaller than I thought. We used to talk about it as an extrapolation problem. And it turns out, guess what, with this much data, they turn into interpolation problems”
Google’s Cultural Blindspot
- DeepMind’s goal was not to build products. DeepMind’s focus was to solve intelligence. It was research.
- It was assumed that eventually this research would accrue value, but no focus was on launching products.
- On the other hand, OpenAI shifted to an approach that both prioritizes 1) long-term research and 2) launching products to consumers and businesses. This resulted in the release of ChatGPT, which was released in November 2022. In response, this marked the beginning of cultural shifts at AI research labs like DeepMind
Matt on changing cultures at AI companies
“I cannot emphasize how much the culture at AI companies, and maybe DeepMind, above all, has changed in the last couple of years. Like we never ever talked about products. It was assumed that once we solved intelligence that was going to be of huge value to Google, like there was a business model, but our day to day life was not about what product can we build and day to day life was very academic. And the question was how do we solve intelligence and so if you think about it from that point of view, training on human data was not solving intelligence. It was just copying intelligence.”
Current State of AI: OpenAI’s first mover advantage potentially fleeting
Google’s Dilemma
Despite cultures shifting away from pure research, Google is still very conservative about what they release publicly because of concerns for public safety and other business stakeholders. Matt believes that Google's tech is much better than the public realizes because of this dynamic. In the short-term, this is an advantage for startups with less “to lose” than Google.
Example: Google’s Music Model
- As an example of this dynamic, Matt mentioned Google has an internal music generation model that outperforms any of the music-generation startups, but that they are not able to release this publicly.
- The model is able to mimic the likeness of artists and generate music in any time structure or style.
- Google believes it is irresponsible to release the model publicly. Specifically, they are concerned that:
- Artists would lose ownership and control of how their voice is used and,
- Music Labels would react negatively out of fear for their business models.
- Instead, they released a series of audio tools for Youtube creators to create and edit music for videos created in combination with Music Labels and artists. The tool lets creators generate short clips using the likeness of artists for their YouTube short clips.
Matt on Google’s music capabilities and why they couldn’t release it
“I said let me hear Elvis Presley singing hound dog, but as a waltz. And Hound Dog is not in ¾ time (Waltz time structure). And it gave me like four different versions of Hound Dog in ¾ time but in a perfect imitation of Elvis Presley's voice. I was like oh my God. This thing understands not only the surface properties of music, like the way that somebody's voice sounds, but it also understands the deep structure of music, like the time signature.
“We can't put that out there. Actually, we shouldn't put it out there. That would be irresponsible. We have a duty to think about things like moral rights. Musicians have a right to the sound of their own voice. It would be irresponsible to put out a tool that allows generation of music that sounds just like your favorite artists.”
Despite OpenAI launching first, Google and Anthropic have caught up and models are comparable.
He points out that a lot of people at Google felt like OpenAI would leave them behind due to their head start on LLMs which would lead them to collecting a lot of user data, consequently refining their models vs. Google’s. However, it is clear that competitors have managed to catch up to OpenAI very quickly - with the latest Gemini (Google) and Claude (Anthropic) models overperforming GPT4 (OpenAI) in many tasks. The two key reasons are the following:
1) it has turned out that data for fine-tuning is just as important -- if not more important -- than pre-training data, and for fine-tuning data quality may be more important than quantity. So just having 'more data' is not automatically the moat that people originally thought it might be.
(2) it has gotten easier and easier to contract for data, if you don't have enough customers to generate it. And gathering data in a targeted way, by contracting for it, can generate higher quality fine-tuning data, too. So there are multiple dimensions and sources for data, making the competition issues more complicated than they at first appeared. But there's no telling how things will play out, going forward, of course.
Matt on OpenAI vs. Google vs. Anthropic
“It wasn't like OpenAI had some sort of special wizardry that was hard to replicate. They were just working on a recipe that the competitors hadn't organized themselves to apply it. It was clear when they (Google, Anthropic, Meta, etc.) decided to focus on it, they caught up and overtook them with Gemini (Google) and Claude (Anthropic) outperforming GPT4 (OpenAI). “
“The models have caught up on standard benchmarks (see image below). But the complicated bit is that performance on those benchmarks doesn't necessarily mean a better user experience "in the wild," or better performance in any specific niche domain. And it doesn't mean that a model that wins on benchmarks AND on user ratings won't fall on its face in some very public way (not thinking of any particular model here, of course;-)

Imperfectness of model benchmarks. Existing benchmarks don’t test models against use cases.
Additionally, Matt spoke about how benchmarks are helpful to compare models - but believes they are still very limiting since they are not evaluating real “jobs” that we want these models to perform. He cautions teams against overfitting to arbitrary benchmarks and to focus on testing their models against the actual use case they are solving for.
Matt on the usefulness of GPT ‘benchmarks’
“Sure, the benchmarks show that Claude and Gemini outperforms GPT4 in some functions. However, I’m not even sure we’re evaluating the stuff that we would really want these systems to do, like, how good a model is to plan a vacation, or design a new factory, etc. The benchmarks are not really testing where we’re pushing the models towards.
“I think there's a real risk that some people working in this space may be falling into the psychological trap where you don't realize [you are not accomplishing your goals] because you're so focused on the benchmarks that you're trying to optimize on. You don't realize there are these other things that you want these systems to do that we haven't even really pressured them to do yet.”
Implications: General purpose models vs. vertical models and vertical applications
First use cases for general AI: Markets where improvements are clear, but imperfection is tolerated.
Although general-purpose models are getting better, Matt believes that domain expertise in specific applications (e.g., legal, customer support) where imperfection is tolerated will see initial adoption. He provided two examples:
Customer Support
Matt is seeing lots of applications in niche areas like Customer Support where LLM based agents are becoming more effective than humans for fraud collection and general-purpose support. He was surprised that these agents were not only more effective in terms of cost, but also in terms of customer satisfaction
“Discovery” in Law
Same for Law on “discovery” of key information for litigation. Historically, you needed to hire an army of interns to sift through hundreds of papers to find the right information during a litigation case. With LLMs, the model can pull and summarize the data much more quickly and cheaply than interns or paralegals. There will still be mistakes, but these can be checked more quickly.
General Models versus Vertical Models and Applications
- Matt believes that as models become more general, they will continue to subsume more specific applications that we thought would have required a fine tuned or vertical model. He shared an example of his son trying to join an AI startup focused on a specific task only for that task to get subsumed by a general LLM forcing the company to pivot
- His intuition is that more important than knowing how to adapt large models to a particular application is the expertise or details of that particular application. More specifically, the UI/UX, the integrations required, the type of data that is important to fine tune correctly, how to interpret that data will all be important to win customers
Example: e-discovery for Law
- Matt notes that Gemini or GPT-4 can likely already handle the task of legal discovery in litigation
- That said, the people at these companies don’t deeply understand the customer or the UI a lawyer needs
- Meaning, general models could handle the task but they might not do it in a way a lawyer needs or in a UI that is built for their workflows and systems. This is an advantage for startups serving vertical applications
- Matt calls out government services as an exception. He notes that Microsoft has a lot of expertise on how to build software for this sector and it’s likely they produce both the model and customers for this set of end customers
Matt on e-discovery and the opportunity for startups
“I’m pretty sure Gemini or GPT can handle the task of discovery in law. But the people working at big labs don't know what the task is. Right? And they don't know how to make a user interface that a lawyer is going to find useful. I think my intuition is it's at that end of service development, where it's going to be very hard for things to get completely absorbed by by the big labs”
Domain expertise that does not exist in labs will be critical for vertical models and applications
- His view is the market is going to bifurcate. We will have enterprise AI tools like Google Cloud and retail assistants that any consumer can use. Neither of these will serve sector specific or use case specific applications like e-discovery for Law or manufacturing in industrial settings. You will need domain experts to build these models and applications for customers.
Example: DeepMind’s AlphaFold (AI for Science)
- The secret sauce in DeepMind’s AI for science research are not AI breakthroughs
- Instead, the secret is DeepMind brought in domain experts (e.g., biologists) to help create the model
- Scientists helped inform the right data sets to use, the right edge cases to focus on, and training to use
- In Matt’s view, domain expertise will continue to be critical for sector specific use cases
Matt on the importance of vertical knowledge
“Re: AlphaFold - The real secret sauce is that Deep Mind brought in some domain experts - actual biologists who were literate enough with the AI tools that they could talk to the ML researchers and guide them on how to train the model - pointing out what are the right data sets, edge cases, etc. and I can't see a near term future where that kind of domain expertise ceases to be important. “
UI/UX will need to be adapted as human behavior and AI systems co-evolve
- Matt believes UI/UX will need to co-evolve with AI systems. As AI’s get more advanced, human behavior and the way we interact with these systems will change. This will impact how a user interacts with a UI/a product.
- Matt used the historical example of Google Fu, which is the skill of optimizing your searches to find information on Google to describe this dynamic. Users will adapt the way they work to maximize their output with AI
- Matthieu Rouif, CEO of Photoroom, noted this dynamic. They started with skeuomorphic design to mimic the existing world and have increasingly introduced new ways of interacting with design/editing as users have gotten used to working with AI applications
Robotics: The potential next breakthrough in AI
- Robotics has not yet had its "GPT moment," but there are signs that this could be the next major breakthrough.
- In the past few months, we’ve seen new robotics startups launching. Matt’s prediction is once robotics reach a similar level of advancements as LLMs, the world’s attention will shift towards robotics.
- He argues the commercial impact and policy impact of robots in the real-world will be broader than language
Matt on the social implications of Robotics
“You think senators are worried about AI now, wait until we have really impressive robotics and you have their constituents freaking out about terminators.”
Implications: Will models scale or do we need new breakthroughs? It’s still unclear.
Lastly - Matt spoke about the downside case of LLMs where LLM performance might hit a plateau and throwing more computers won’t improve model performance. This would lead to slower progress and another AI winter. Matt noted that this has happened before and there is no reason it can’t happen again. In the scenario, where AI continues to scale and systems become more general, he believes it will become even more important to understand your end customer, their workflow, and their use case deeply. Otherwise, business value will accrue to general labs.
Matt on the uncertainty of AGI timeline
“AI has had many ‘winters’ when things plateaued. There's no reason why that can't happen again, like absolutely no reason. And if that happens, then there's going to remain this like, very vibrant sector of people fine tuning and adapting, and we'll kind of stay where we are right now, in terms of the business opportunities. If the ceiling turns out to be much higher, and these systems end up being out of the box applicable to a much wider range of applications, then I think the business opportunity for people outside the big Labs is going to get narrower and narrower, right? It's going to be like really knowing the customer right in a way that the big labs don't.”
Comments